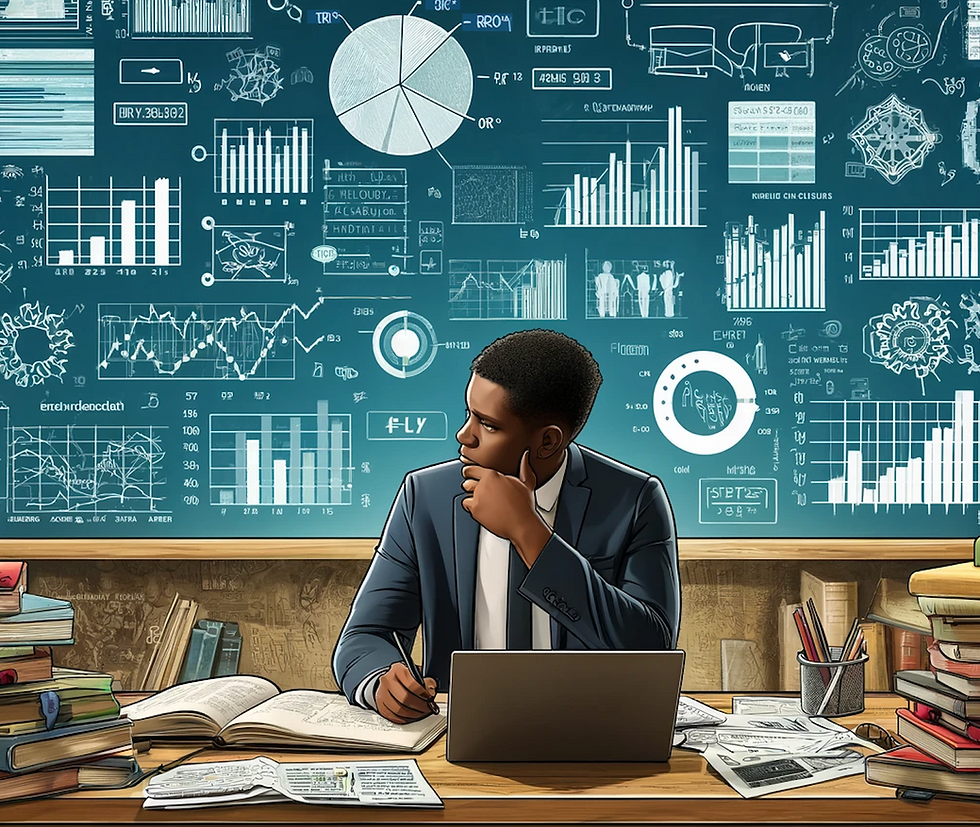
In today's data-driven society, it's often assumed that numbers can tell us everything we need to know—especially in sectors like education, workforce, and community development. While data is crucial for identifying disparities and measuring progress, it alone cannot capture the full spectrum of social, economic, and cultural factors that influence outcomes.
But the catchy phrase, "the numbers speak for themselves," is not only misleading, it's actually harmful.
Operating as though "the numbers speak for themselves" shows a misplaced confidence in the clarity and objectivity of data as though the data alone can fully explain complex social equity issues such as education and workforce development. Data often overlooks the nuanced realities of systemic inequalities and individual experiences that are essential for understanding and addressing these issues effectively.
The Limitations of Relying Only on Data
At Opportunity Consulting, data helps illuminate where disparities exist and track changes over time. Data is also a starting place for our work when looking to make sense of the realities in systems and communities because it provides a baseline of outcomes.
Yet, data alone does nothing to uncover the real world issues that create these gaps.
For instance, in education, data showing lower test scores for certain demographic groups can lead to quick assumptions about capabilities without considering underlying factors such as access to resources, quality of learning, high churn rates in some schools, and safety conditions. Similarly, in communities and the workforce, data might show lower rates of homeownership or higher unemployment in certain areas, but without understanding the systemic barriers like historic redlining or economic disinvestment, devised solutions may be ineffective because they are actually intended to solve the wrong problem.
A More Thoughtful Approach to Data
Adopting a more thoughtful approach to data involves recognizing and addressing its inherent limitations and biases. This approach (called QuantCrit) provides a framework for understanding how data reflects broader societal inequities and helps to challenge oppressive structures within data narratives.
By integrating some key tenets into our work, we aim to foster needs assessments and plans, program designs, and strategic initiatives that are not only done through an equity-lens and informed by data but also critically aware of the contexts and power dynamics that shape that data.
Recognize Bias in Data: Data is not collected or interpreted in a vacuum. It can reflect the biases of those who collect and analyze it, potentially leading to skewed results, biased messaging, and incorrect conclusions. Researchers often fail to adequately discuss these biases, leading many to interpret quantitative findings as objective facts. Our work should start by acknowledging that all data and analysis methods introduce biases and strive to minimize and explicitly discuss these biases.
Ask: Who collected this data? What assumptions may have influenced its collection and interpretation?
Consider: How might these biases impact our understanding and the solutions we propose?
Question Our Categories…. Psssst: The Numbers are not Neutral or Natural: The groups we use to sort data, such as race, income levels, or educational attainment, are constructed by society. They can unintentionally perpetuate stereotypes or obscure important nuances. We should view these categories as neither natural nor inherent, understanding that they are socially constructed and fluid.
Seek Out and Listen to Those Represented in the Data: Data should always be complemented with insights from the people it represents. Engaging directly with community members can provide context and depth that quantitative data alone cannot offer. We should emphasize that data cannot speak for itself and requires the perspective of those it represents to ensure that interpretations do not reinforce existing deficit narratives about minoritized groups.
People are Complex... So Attend to the Intersectionalities in the Data: People's lives and experiences are not one-dimensional. They’re shaped by multiple, intersecting factors—race, class, gender, geography, learning and thinking differences, etc. Understanding these intersections is crucial for a thorough analysis. Taking an intersectional perspective allows us to explore how these different aspects of identity interact and affect individual experiences.
Value Narrative and Counter-Narrative: We place great value on individuals' lived experiences, captured through narratives and counter-narratives. These counter-narratives, which often contradict dominant societal norms or beliefs, are crucial for understanding the experiences of minoritized or marginalized individuals.
Our Outcome-Led Equity(™) Approach to Research and Policy
Our approach to equity-driven work with school districts, workforce systems, and municipalities is structured around four core principles.
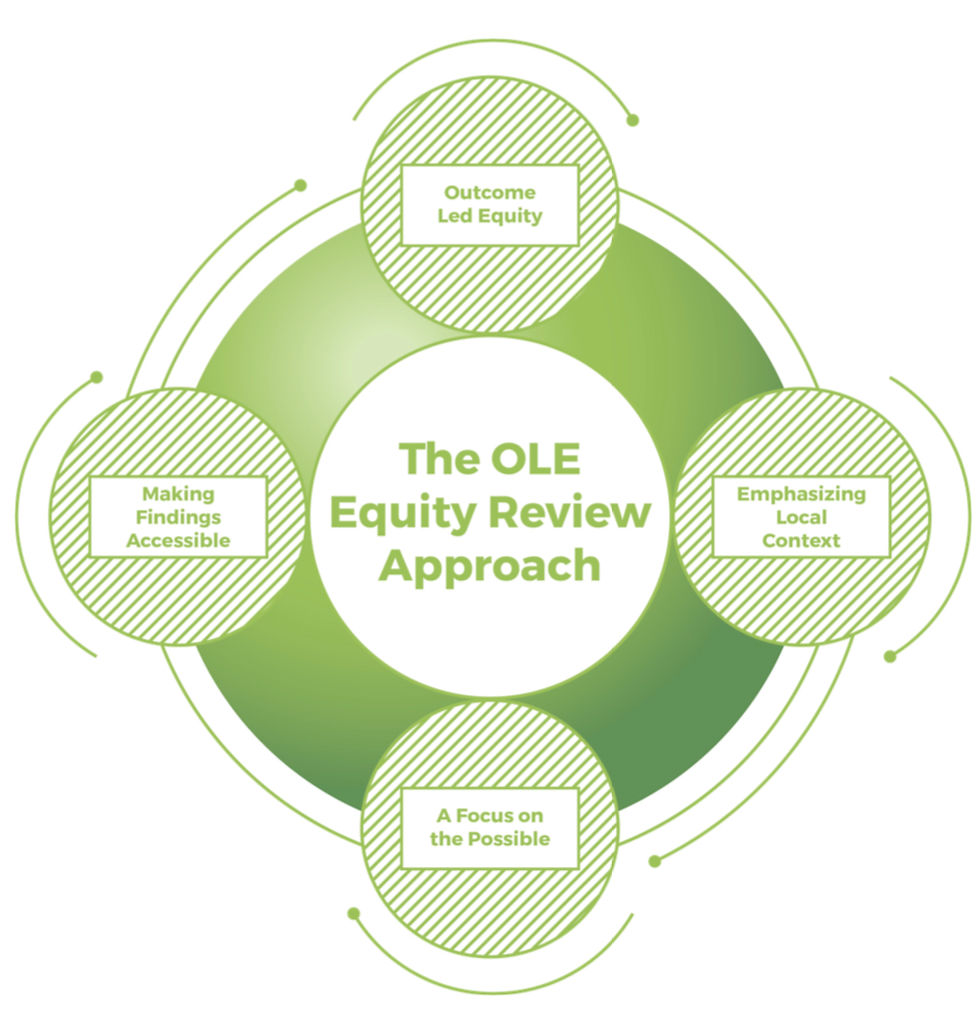
Outcome-Led: We start with data and use the tenets above to interrogate and visualize the data for clear, actionable outcomes that guide our work. This ensures that our efforts are purposeful and directly linked to achieving specific improvements.
Stakeholder-Centered: We prioritize the involvement of all relevant stakeholders—job seekers, students, parents, educators, local residents, business owners/employers, etc.— not just in the beginning but at pivotal points throughout so they’re informing the analysis and solution-development processes.
Focus on the Possible: Our approach is optimistic while we set practical and achievable goals, backed by policy and community support, to ensure sustainability is at the forefront.
Accessible to All: What good is exquisite data and research if it's not accessible to those who need to know it to hold leaders accountable? We aim to translate complex information into clear insights and use storytelling to compel action.
A Personal PSA on Using "Subgroup" Language and Representation in Data
In our efforts to promote equity, we offer a language shift for all who represent people in data to consider: Please remove "sub" from categories of groups.
Traditionally, some groups have been labeled as "subgroups," implicitly suggesting a hierarchy where certain groups are considered the norm and others are deviations from that norm. To disrupt this deficit language and the othering it perpetuates, we refer to all groups simply as "student groups" or "community groups," and label them by specific identifiers such as race or other relevant factors.
These groups are treated as equals in our analyses and representations, ensuring that no one group is considered a baseline or norm against which others are measured. This approach respects the diversity of experiences and conditions within each group and avoids perpetuating a racialized or “othering” perspective in our work.
Conclusion
While data is invaluable in measuring equity in education, workforce, and community development, it must be approached with a critical eye and a commitment to inclusivity.
By combining quantitative data with qualitative research and extensive community engagement, we can create a more accurate and comprehensive understanding of the challenges faced by these communities.
This integrated approach not only reveals the limitations of data but also empowers us to develop solutions that support and uplift entire communities, leading to sustainable improvement and growth. Through mindful engagement with data and a commitment to equitable language and representation, we pave the way for more just and effective policy-making and community support.
Comentarios